Analyzing Emotions in Politics: An In-Depth Look at the RTL-Debate
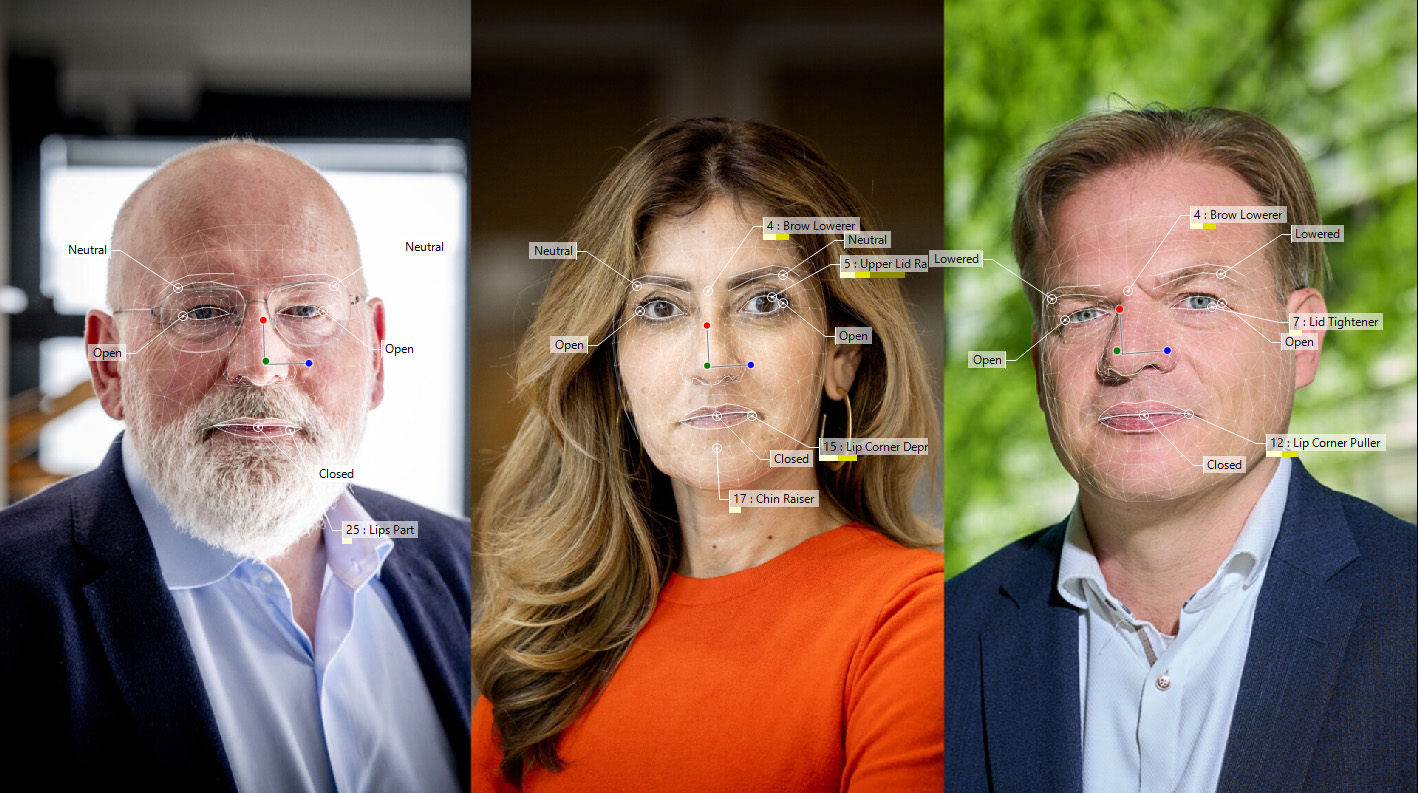
Never before has the Dutch electorate seen so many televised debates as in the run-up to the coming parliamentary elections: three on NOS, two on RTL, and one on SBS. Compared to other in-depth political content, these debates attract relatively large audiences, usually well over a million viewers.
It is well known that votes are not won solely with substantive arguments. Rhetoric, body language, tone of voice and other aspects can attract or deter potential voters. A great example is the Nixon vs. Kennedy debate. Kennedy was considered the clear winner by the television audience, credited to his calm demeanor compared to the sweating and twitching Nixon. However, the radio audience concluded Nixon to be the winner of the debate, as his powerful voice and strong message gave him the upper hand.
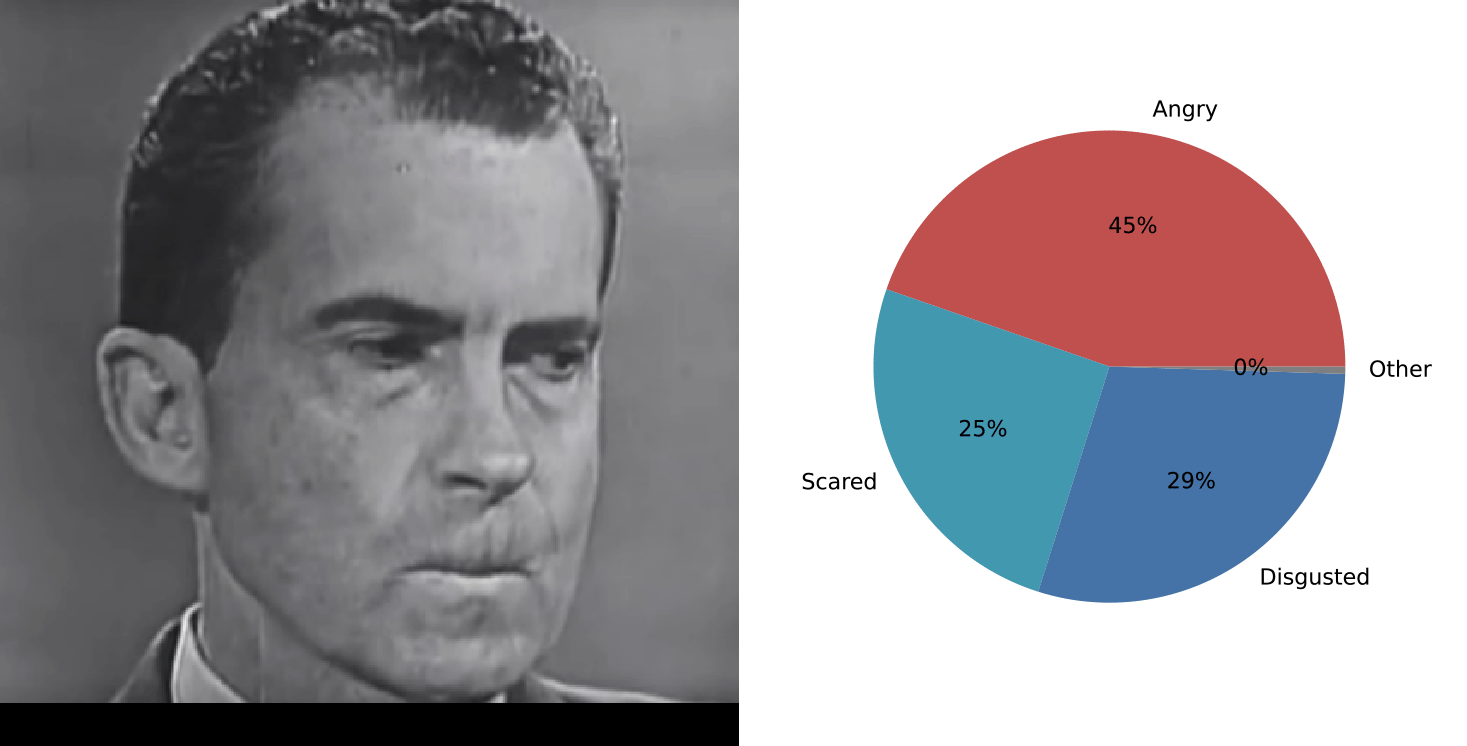
Research indicates that the expression of emotion is multimodal and that several distinct expressions frequently have a typical expression in the voice and face (but also e.g. touch, see chapter 27). At VicarVision, we have a long history of analyzing emotions using facial cues, and we have done this before in political communication. We have previously conducted analyses in political communication, such as during the elections in the US (2012) and Turkey (2014). More recently, we have initiated the development of Speech Emotion Recognition (SER), which analyzes emotions present in the tone of voice of speakers. In the past few years, this technology has reached a level of maturity where, for instance, investors are analyzing executives’ promises.
Emotional analysis of candidates
We used both facial cues and SER to analyze the first RTL debate, featuring the leaders of the three biggest parties: Frans Timmermans (GroenLinks-PvdA), Dilan Yeşilgöz (VVD), and Pieter Omtzigt (NSC). We split the results by the various electoral issues discussed during the debate, offering insights on which themes they – consciously or unconsciously – show specific emotional responses.
When looking at the general emotional responsivity – what ratio of speech is emotional rather than neutral – we can see that all speakers show a ratio of about 20% to 45% (left figure). However, if we look at specific emotions, the differences become much clearer. For instance, when looking at anger, Timmermans displays this emotion when discussing the decline in standard of living, while Omtzigt shows discontent towards the leadership of the past years (right figure).”
Speaker analysis
Pieter Omtzigt: Confident and optimistic
Pieter Omtzigt appeared overwhelmingly happy, likely due to his strong confidence stemming from high poll rankings and a clear grasp of his key talking points. He exhibited optimism, especially when discussing the economy and standard of living. However, a noticeable shift to anger was observed when the conversation turned to political leadership and integrity, highlighting his strong stance on these issues.
Dilan Yeşilgöz: Complexity of Emotions
Dilan Yeşilgöz exhibited a complex emotional palette. She oscillated between happiness and sadness when discussing climate change, standard of living and leadership. Her emotional response during debates on international crises – which was discussed during the ‘leadership’ topic – was particularly marked by sadness, suggesting an empathetic attitude towards public hardships.
Frans Timmermans: Passionate and Steadfast
Frans Timmermans stood out for his significant display of anger, especially on topics like standard of living, the minimum wage, and the housing crisis. This passionate response likely indicates a deep commitment to addressing these critical issues. His relatively steady emotional tone in other areas suggested a composed approach to the debates’ various topics.
Reactions to other speakers
Beyond the evident advantage of multimodality, which significantly improves the accuracy of predicting emotions by integrating both vocal tones and facial expressions, it also allows us to observe how individuals express themselves through facial cues while others are speaking. The figure below presents each participant’s facial expressions in rows, with the columns denoting the speaker. For example, it demonstrates that Omtzigt predominantly exhibits anger when he speaks, whereas he shows less anger when listening to others (bottom row). Additionally, Timmermans displays the strongest expression of anger when Omtzigt is speaking (top-right), while Omtzigt appears most cheerful when Yeşilgöz is speaking (bottom-center). This observation could suggest Omtzigt’s leaning towards a coalition with Yeşilgöz rather than Timmermans the following day.
The diagonal (top-left to bottom-right) indicates the facial expressions of the subjects while they are speaking themselves.
Conclusion
While speech emotion recognition algorithms measure expressed emotions rather than an individual’s actual underlying emotional state, they still offer valuable insights into the priorities and values. This becomes evident in instances such as Timmermans’ display of anger concerning social issues or Omtzigt’s frustration with political leadership. This analysis takes a broad perspective to showcase the correlations between topics and basic emotions. Using basic emotion theory is only one way of analysing emotions (next to e.g. dimensional. appraisal theories), and more detail and subtleties could be discovered by using for example, smaller activations in the face (e.g. by using action units). See this blog on different scientific theories on emotion for a more elaborate description on how to use FaceReader within different theoretical approaches.
All in all, this analysis demonstrates the potential power of emotion recognition technology in comprehending the intricacies of political debates. Given the pivotal role emotions play in shaping voter opinions, this technology has the potential to significantly impact the formulation of political strategies and the way candidates connect with the electorate.